Navigating AI Risk Management: Your Framework for Success
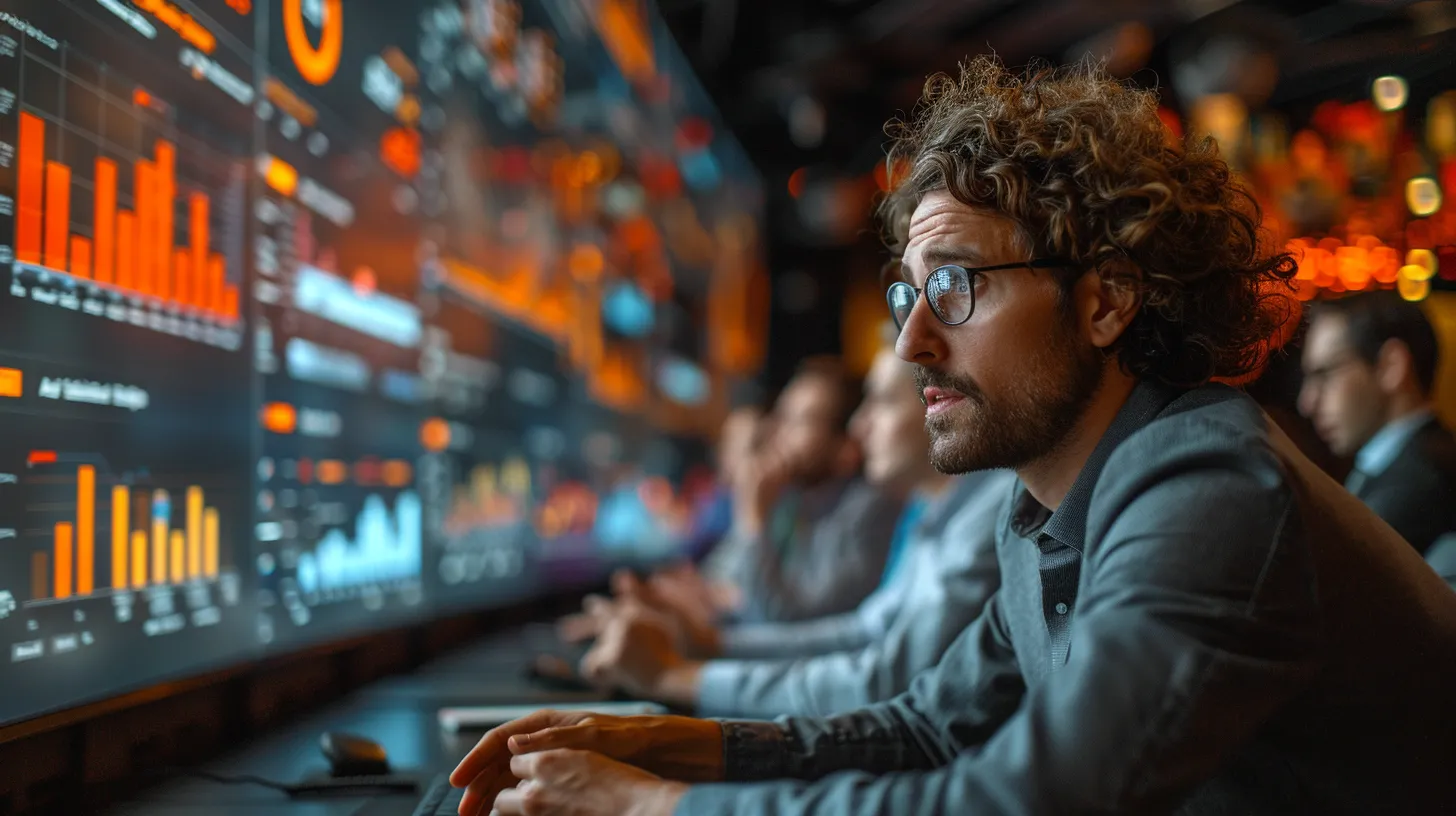
Our audience supports Ahcrypto. When you click on the links on our site, we may earn an affiliate commission at no extra cost to you. Learn More.
Understanding AI Risk Management
Artificial Intelligence (AI) risk management encompasses the strategies and processes implemented to identify, assess, and mitigate risks associated with AI systems. The framework for addressing AI risks is crucial in ensuring the reliability and security of AI technologies.
Key Takeaways
Overview of AI Risk
Understanding the risks associated with AI is essential to manage its deployment effectively. AI risk refers to the potential threats and vulnerabilities that AI systems may pose, including cybersecurity breaches, reputational damage, and data misuse.
Stay Updated with the Latest Digital Marketing Tips!
Subscribe to our newsletter and receive our exclusive guide, “Top 10 Digital Marketing Strategies for Success,” straight to your inbox
Importance of AI Risk Management
The importance of AI risk management cannot be overstated as organizations increasingly rely on AI technologies. Proper risk management frameworks help mitigate potential risks, ensure regulation compliance, and build stakeholder trust.
Critical Components of AI Risk Management
Critical components of AI risk management include governance structures for overseeing the development and use of AI, risk assessment methodologies, compliance with standards such as NIST’s AI Risk Management Framework, and implementing best practices to evaluate and govern AI systems.
Navigating AI Risk Management: Your Framework for Success
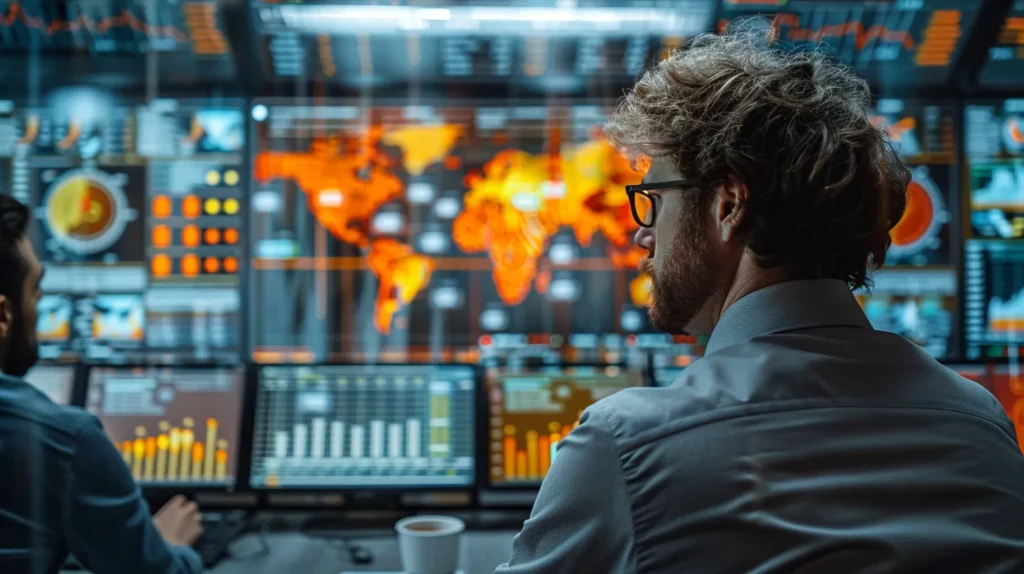
Navigating AI Risk Management: Your Framework for Success begins with understanding the definition of AI and its diverse applications. Developing a robust Artificial Intelligence Risk Management Framework is crucial for assessing AI risk throughout the lifecycle of an AI system.
Incorporating AI governance and AI standards, such as those anticipated in the European Union’s upcoming AI Act, helps organizations ensure that their AI tools are practical and aligned with AI regulations. This approach not only supports responsible AI but also prepares organizations to understand and mitigate the risks of AI, including the potential for bias in AI.
Developing a playbook based on the NIST AI RMF Playbook can provide a step-by-step guide to operationalize these principles. Essential elements include selecting AI use cases, managing training data to ensure quality and minimize bias, and continuously monitoring how well an AI model performs in a real-world AI environment. Tailoring risk management and mitigation strategies to specific types of AI, such as generative AI, is vital for innovation and safety, driving towards trustworthy AI that meets the expectations of a forthcoming AI Bill of Rights.
Successful AI efforts require a dynamic approach to risk analysis and understanding the unique challenges of different applications, from simple AI services to complex AI systems. Organizations can navigate the complexities of artificial intelligence risk management by focusing on responsible AI practices and aligning with global efforts for AI governance, such as the European Union’s guideposts. Utilizing specific frameworks and guidelines will help organizations use AI more effectively and create a more trustworthy AI landscape.
Understanding AI Risk Management
Artificial Intelligence (AI) risk management involves implementing strategies and processes to identify, assess, and mitigate risks linked with AI systems. Establishing a robust framework is crucial for ensuring the dependability and security of AI technologies.
Overview of AI Risk
Recognizing AI risks is fundamental to managing its implementation effectively. AI risk involves potential threats and vulnerabilities such as cybersecurity breaches, reputational harm, and data misuse that AI systems could encounter.
Importance of AI Risk Management
Given the growing dependence on AI technologies, AI risk management is paramount. Comprehensive risk management frameworks help reduce potential risks, ensure regulatory compliance, and foster stakeholder trust.
Critical Components of AI Risk Management
Essential elements of AI risk management include governance structures overseeing AI development and usage, risk assessment methodologies, adherence to standards like NIST’s AI Risk Management Framework, and the incorporation of best practices for evaluating and governing AI systems.
Implementing a Risk Management Framework
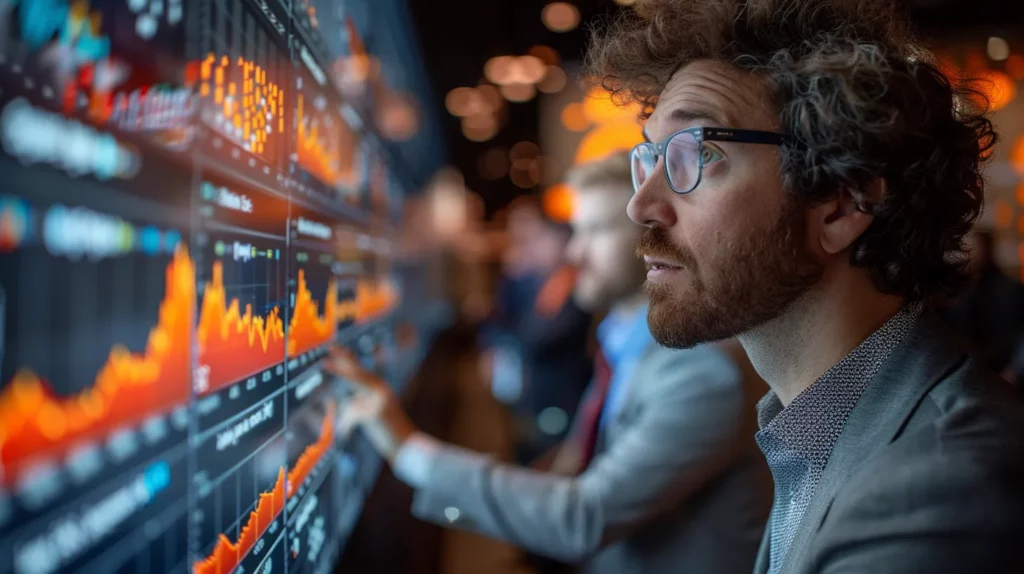
Establishing a reliable risk management framework is essential for organizations aiming to deploy AI systems effectively. By implementing the National Institute of Standards and Technology (NIST) guidelines, organizations can ensure comprehensive risk management tailored to AI technologies.
Role of NIST Guidelines
The NIST guidelines play a crucial role in shaping AI risk management strategies. They provide a structured approach to addressing AI risks and offer valuable insights into risk mitigation, compliance standards, and best practices for managing AI risks effectively.
Developing an AI Risk Management Framework
Developing a tailored AI risk management framework is a strategic endeavor for organizations. By customizing risk management processes to the specific risks associated with AI systems, organizations can proactively enhance their ability to assess, mitigate, and respond to AI-related risks.
Integrating AI Risk Management into Existing Systems
Integrating AI risk management practices into existing systems ensures a holistic approach to risk mitigation. By embedding AI risk management processes into the lifecycle of AI technologies, organizations can enhance cybersecurity measures, regulatory compliance, and overall risk resilience.
Artificial Intelligence (AI) risk management involves implementing strategies and processes to identify, assess, and mitigate risks linked with AI systems. Establishing a robust framework is crucial for ensuring the dependability and security of AI technologies.
Overview of AI Risk
Recognizing AI risks is fundamental to managing its implementation effectively. AI risk involves potential threats and vulnerabilities such as cybersecurity breaches, reputational harm, and data misuse that AI systems could encounter.
Importance of AI Risk Management
Given the growing dependence on AI technologies, AI risk management is paramount. Comprehensive risk management frameworks help reduce potential risks, ensure regulatory compliance, and foster stakeholder trust.
Critical Components of AI Risk Management
Essential elements of AI risk management include governance structures overseeing AI development and usage, risk assessment methodologies, adherence to standards like NIST’s AI Risk Management Framework, and incorporating best practices for evaluating and governance.
Auditing and Assessing AI Systems
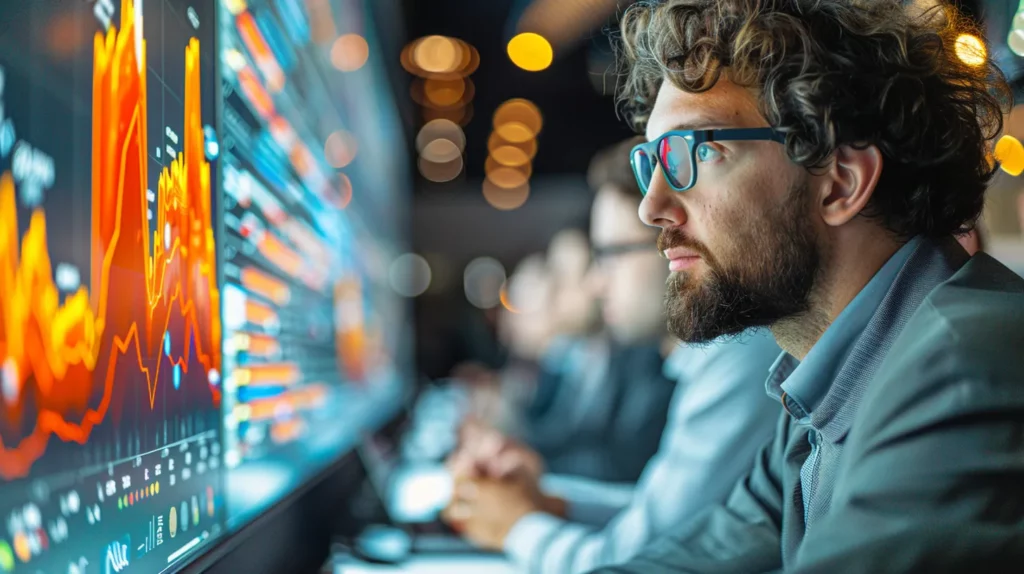
Implementing thorough auditing processes is essential to ensure the effectiveness and security of AI systems. Auditing involves evaluating AI systems’ algorithms, data sets, and methods to identify vulnerabilities and compliance issues.
Conducting AI System Audits
Regular audits of AI systems help to assess their performance, accuracy, and adherence to regulatory standards. Organizations can identify areas for improvement and address potential risks proactively through audits.
Evaluating High-Risk AI Applications
Evaluating high-risk AI applications involves analyzing the potential impact of AI systems on business operations, data privacy, and ethical considerations. Thorough evaluations can help organizations prioritize risk mitigation strategies for critical AI applications.
Compliance with NIST AI RMF
Ensuring compliance with the NIST AI Risk Management Framework is crucial for aligning AI risk management practices with industry standards. Compliance with NIST guidelines helps establish a structured approach to managing risks associated with AI technologies.
Enhancing Risk Management Practices
Continuously monitoring and evaluating AI systems is essential for proactively managing risks. By enhancing risk management practices, organizations can adapt to the evolving AI landscape and strengthen their resilience against emerging threats.
Continuous Monitoring and Evaluation
Continuous monitoring and evaluation of AI systems help identify risk profile changes and ensure the effectiveness of risk mitigation strategies. Organizations can detect and address potential vulnerabilities by continuously assessing AI systems.
Addressing Core Functions within AI Risk Management
Addressing core functions within AI risk management involves aligning risk mitigation strategies with AI systems’ specific risk profiles. Organizations must focus on critical areas such as data governance, algorithm transparency, and cybersecurity measures to enhance their risk management practices.
Staying Updated on the Evolving AI Risk Landscape
Staying informed about the evolving AI risk landscape is essential for proactively adapting risk management practices. By staying updated on emerging threats, regulations, and best practices, organizations can enhance their ability to effectively mitigate risks and ensure the trustworthy deployment of AI technologies.
Conclusion
In conclusion, effectively navigating AI risk management is crucial for the safe and responsible deployment of AI technologies. Organizations can mitigate the risks associated with AI by incorporating comprehensive frameworks, such as integrating AI governance, adhering to standards like the AI Act, and implementing thorough risk assessment methods. Emphasizing the importance of trustworthy AI, adherence to regulatory requirements, and continuous monitoring ensures that AI systems are practical and ethically aligned. As we advance, staying informed and adaptable to evolving AI landscapes will be vital to harnessing the benefits of AI while safeguarding against its potential risks.
Keep updated on all of our latest tips here.
FAQ
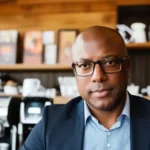
Scott Evans
Hey there, I’m Scott Evans, your friendly guide at AhCrypto! I’m all about breaking down complex SaaS, AI, and tech topics into digestible insights. With me, you’re not just keeping up with the tech world; you’re staying ahead of the curve. Ready to dive into this exciting journey? Let’s get started!